Reflecting on Consent at Scale
By Leah Ajmani and zentx005 on
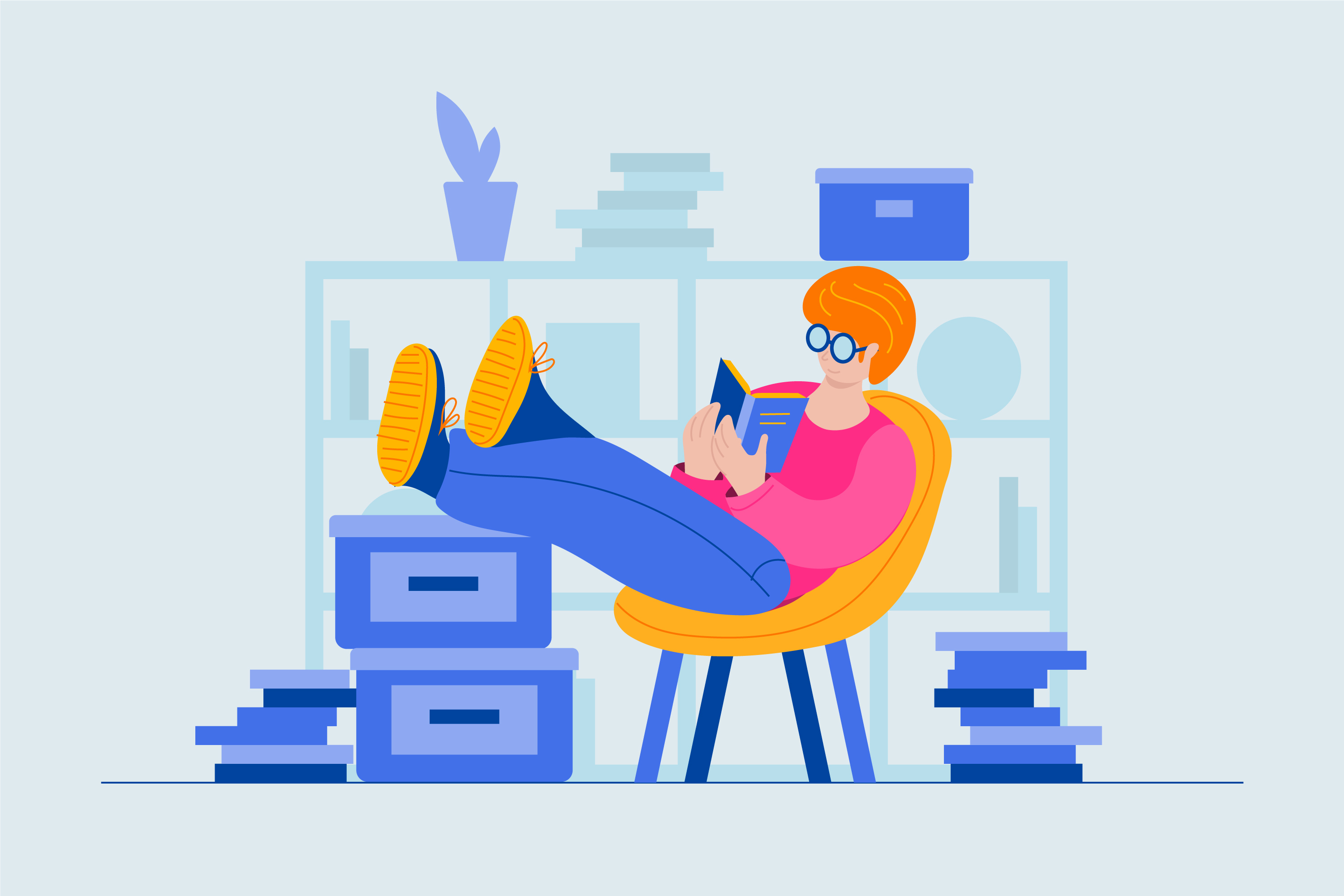
image by Freepik
In the era of internet research, everyone is a participant. Picture this…
A PhD stood at the front of a crowded conference hall. They’d just presented their paper on social capital in distributed online communities. As the applause settled, an audience member scuttled to the microphone, eager to ask the first question.
A professor from University College. Thank you for the great talk. It was refreshing to attend a talk with such rigorous methods. You scrapped data from so many different subreddits and made such a compelling argument for how these results will generalize to other online spaces. My question is less about the research and more about your experiences with data contributors. How did the various subreddit community members react when you talked to them about this exciting work?
What kind of question is this? The PhD thinks to themself. It’s not feasible to get consent from every user. We got an IRB exemption, got approval from subreddit moderators, and followed all the API terms of use and regulations for researcher access. Do other researchers really ask for consent at scale? Did I get consent…?
You may be in a similar situation now! Using social media data for research is a common method that has massive potential for large-scale analyses in both quantitative and qualitative research. However, it can be frustrating to simultaneously hold individual, affirmative consent as the golden standard and recognize its limitations as a viable option for many researchers. To that end, we’ve made a reading list about getting individual consent at scale, particularly in research settings. We hope this reading list serves as a provocation for discussion rather than a list of solutions to this problem.
Normative Papers
1. The “Ought-Is” Problem: An Implementation Science Framework for Translating Ethical Norms into Practice. Our resident ethicist (Leah Ajmani) loves this paper so much! It basically uses informed consent as a case to describe the larger translational effort needed to move from normative prescriptions to actual implementation.
2. Yes: Affirmative Consent as a Theoretical Framework for Understanding and Imagining Social Platforms. A contemporary classic in CHI, this paper does a really good job of describing affirmative consent as the ideal situation but then using the “ideal” for explanatory and generative purposes. There is merit to having an ideal, even if it is not perfectly attainable!
HCML Papers
We’re obviously biased because she’s a GroupLenser, but Stevie Chancellor does a great job at describing consent at scale as an ethical tension rather than a “must-have.” It is something researchers need to navigate with justified reasoning.
1. A Taxonomy of Ethical Tensions in Inferring Mental Health States from Social Media
2. Toward Practices for Human-Centered Machine Learning
Design Papers
These papers are both critical of current consent design and do a great job of discussing alternatives, even if it is outside of a research context.
1. (Un)informed Consent: Studying GDPR Consent Notices in the Field
2. Limits of Individual Consent and Models of Distributed Consent in Online Social Networks
From grappling with moral nuance to designing better consent procedures, these readings can take our discussions of individual consent at scale from a theoretical ideal to an operationalizable goal. So, let’s embrace difficult discourse about how to move forward and continue to traverse the space between the idyllic and the feasible. Comment or tweet which papers you would add to this list!