What even is AI justice? Proposing a Theory of Justice at FAccT 24
By Leah Ajmani on
In 2022, Politico reported that Crisis Text Line (CTL)–a non-profit SMS suicide hotline–-used one-on-one crisis conversations to train a for-profit customer service chatbot. In response, CTL maintained that they did not violate reasonable expectations—hotline users “consented” to a lengthy Terms of Service (TOS), which specified that CTL could use data for business purposes.
This uninformed consent procedure and irresponsible use of data is an obviously egregious move in many research circles, such as my own. However, in the eyes of the law, CTL, and many others, CTL didn’t violate any reasonable user expectations. The consensus was that, while TOS consent procedures are imperfect, it’s the cost of having free technology services. In my paper, I ask: are we still okay with paying this price?
The price here is justice—our ability to give everyone their due right. In offline settings, injustice evokes the image of human rights violations and massive inequities. However, the picture is less clear in AI settings. As we all contribute our data, knowledge, and time to AI systems, what do we deserve as a matter of justice? To answer this question, we formulated a precise theory of justice that captures current tensions between users and tech companies in AI/ML settings.
To quote John Rawls,
“A theory however elegant and economical must be rejected or revised if it is untrue; likewise laws and institutions no matter how efficient and well-arranged must be reformed or abolished if they are unjust.”
In other words, a theory of justice is an attempt to appropriately represent the state of the world, articulate societal values, and inform future change. When we have a true theory of justice, we do not need to rely on subjective moral intuitions. Rather, we can agree upon an ethical compass for building our laws and institutions.
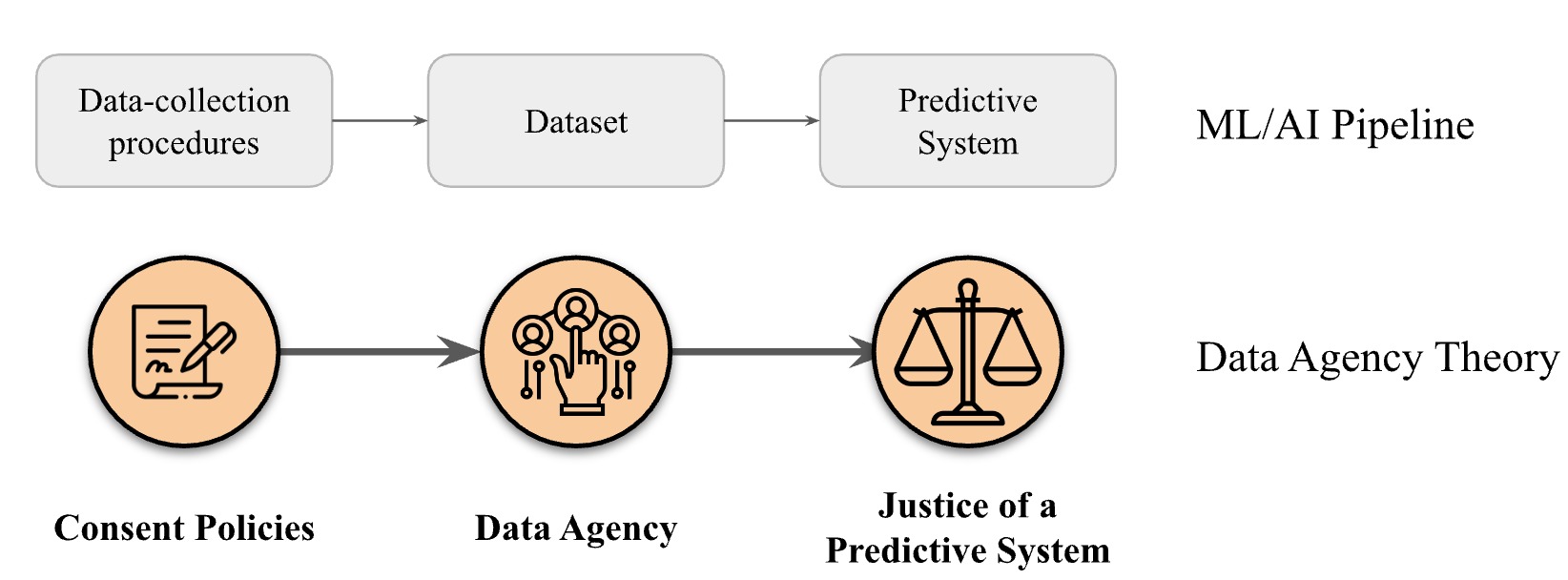
Our paper proposes data agency theory (DAT). Data agency is one’s capacity to shape action around the data they create. For example, individual privacy settings on Google increase one’s data agency by allowing users to opt into (i.e., consent to) data sharing with third-party advertisers. Data agency theory argues two premises. First, consent procedures outline data agency systematically and, therefore, are institutional. Second, inspired by justice scholars such as Rawls and Young, this institutional way of outlining data agency is a matter of justice. In sum, justice in a predictive system demands considering how institutional routines (i.e., consent procedures and terms of services) transform agency at a group level. Concisely, data agency is a contributor to justice and a product of consent policies in a predictive system.
DAT is a data-centric theory of justice that directly translates to next steps for achieving ML & AI ethics goals. As ML/AI systems need larger datasets, ethicists in the field have made numerous calls for better data management throughout the pipeline—involving questions of consent, data storage, and responsible use of predictive outcomes. However, these calls have been mostly unanswered; many social media sites still use dense Terms of Service agreements that have been criticized for over a decade. Here, we argue that this lack of action to increase a user’s data agency is not just a moral imperfection, it is injustice.
By raising the stakes of problematic consent procedures, we hope to catalyze action. In the paper, we reimagine consent procedures in two salient ML/AI data contexts: (1) social media sites and (2) human subjects research projects. For example, we imagine affirmative consent on social media sites, sustained efforts by researchers to reaffirm consent, and the ability to withdraw one’s data from benchmark datasets. AI justice demands consent procedures that proactively solve systemic information and power gaps around one’s data. This paradigm shift is crucial to evaluating current consent procedures and generating better ones.
What does a just world with AI look like? How can we evaluate the justice of our AI systems before they cause material harm? Check out our paper for discussions of these questions, or come to my talk at FAccT during the Towards Better Data Practices session at 3:45pm (UTC-3) on June 4th.