I sat in a gray cubicle, next to a social worker deciding whether to investigate a young couple reported for allegedly neglecting their one-year-old child. The social worker read a report aloud from their computer screen: “A family member called yesterday and said they went to the house two days ago at 5pm and it was filthy, sink full of dishes, food on the floor, mom and dad are using cocaine, and they left their son unsupervised in the middle of the day. Their medical and criminal records show they had problems with drugs in the past. But, when we sent someone out to check it out, the house was clean, mom was one-year sober and staying home full-time, and dad was working. But, dad said he was using again recently.” The social worker scrolled down past the report and clicked a button; a screen popped up with “Allegheny Family Screening Tool” at the top and a bright red, yellow, and green thermometer in the middle. “The algorithm says it’s high risk.” The social worker decided to investigate the family.
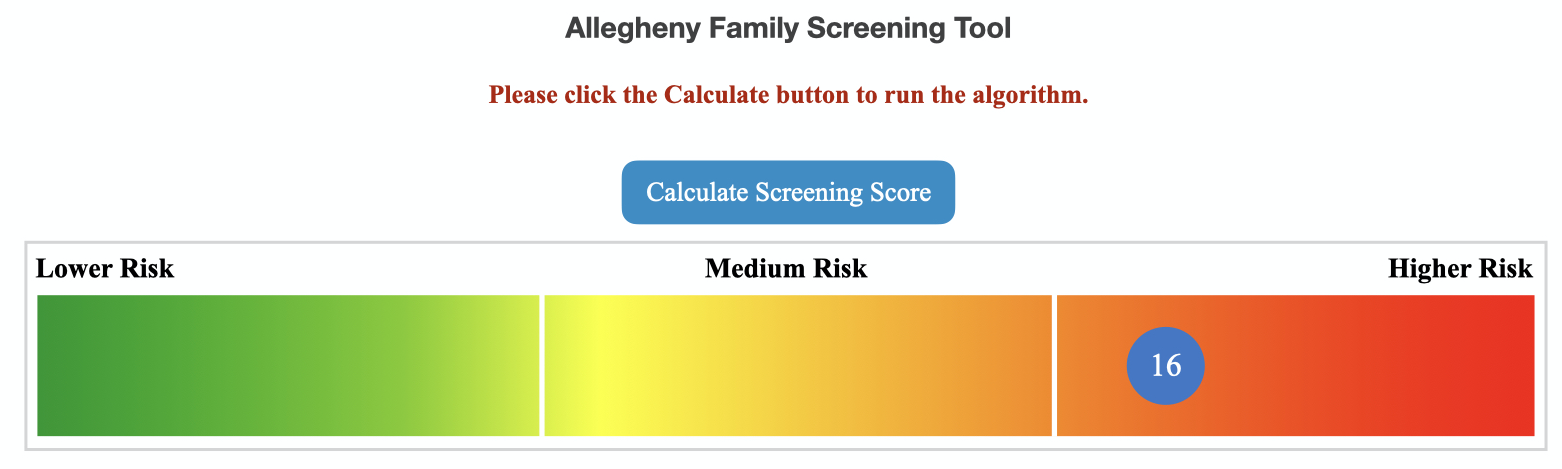
Workers in Allegheny County’s Office of Children, Youth, and Families (CYF) have been making decisions about which families to investigate with the Allegheny Family Screening Tool (AFST), a machine learning algorithm which uses county data including demographics, criminal records, public medical records, and past CYF reports to try to predict which families will harm their children. These decisions are high-stakes: An unwarranted Child Protective Services (CPS) investigation can be intrusive and damaging to a family, as any parent of a trans child in Texas could tell you now. Investigations are also racially disparate: Over half of all Black children in the U.S. are subjected to a CPS investigation, twice the proportion for white children. One big reason why Allegheny County CYF started using the AFST in 2016 was to reduce racial biases. In our paper, How Child Welfare Workers Reduce Racial Disparities in Algorithmic Decisions, and its associated Extended Analysis, we find that the AFST gave more racially disparate recommendations than workers. In numbers, if the AFST fully automated the decision-making process, 68% of Black children would’ve been investigated and only 50% of white children from August 2016 to May 2018, an 18% disparity. The process isn’t fully automated though: the AFST gives workers a recommendation, and the workers make the final decision. Over that same time period from 2016 to 2018, workers (using the algorithm) decided to investigate 50% of Black children and 43% of white children, a lesser 7% disparity.
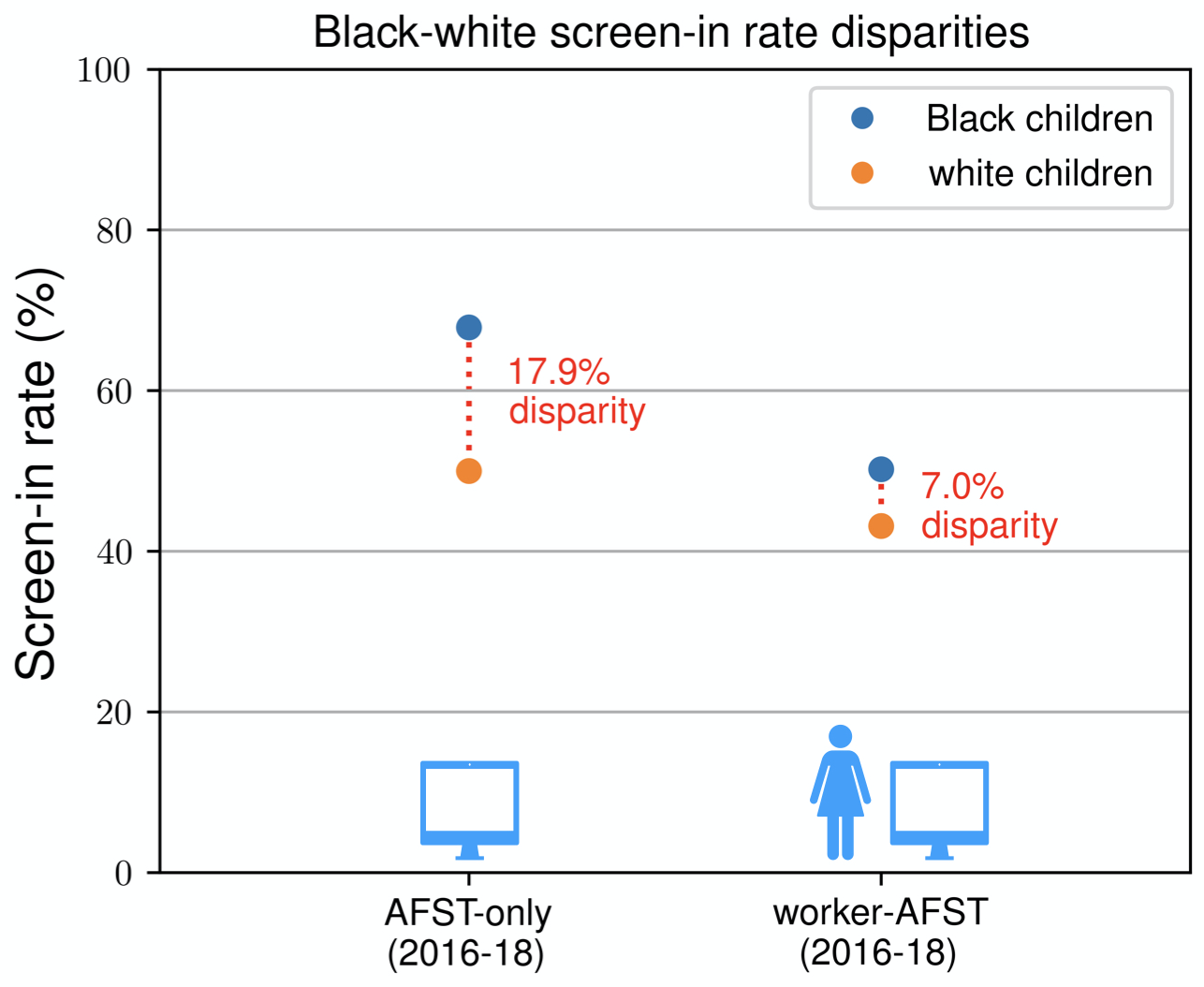
This complicates the current narrative about racial biases and the AFST. A 2019 study found that the disparity between the proportions of Black and white children investigated by Allegheny County CYF fell from 9% before the use of the AFST to 7% after it. Based on this, CYF said that the AFST caused workers to make less racially disparate decisions. Following these early “successes,” CPS agencies across the U.S. have started using algorithms just like the AFST. But, how does an algorithm giving more disparate recommendations cause workers to make less disparate decisions?
Last July, my co-authors and I visited workers who use the AFST to ask them this question. We showed them the figure above and explained how the algorithm gave more disparate recommendations and that they reduced those disparities in their final decisions. They weren’t surprised. Although the algorithm doesn’t use race as a variable, most workers thought the algorithm was racially biased because they thought it uses variables that are correlated with race. Based on their everyday interactions with the algorithm, workers thought it often scored people too high if they had a lot of “system involvement,” e.g. past CYF reports, criminal records, or public medical history. One worker said, “if you’re poor and you’re on welfare, you’re gonna score higher than a comparable family who has private insurance.” Workers thought this was related to race because Black families often have more system involvement than whites.
The primary way workers thought they reduced racial disparities in the AFST was by counteracting these patterns of over-scoring based on system involvement. A few workers we talked with said they made a conscious effort to reduce systemic racial disparities. Most, however, said reducing disparities was an unintentional side effect of making decisions holistically and contextually: Workers often looked at parents’ records to piece together the situation, rather than as an automatic strike against the family. For example, in the report I mentioned at the top of this article, the worker looked at criminal and medical records only to see if there was evidence that the parents abused drugs. The worker said, “somebody who was in prison 10 years ago has nothing to do with what’s going on today.” Whether they acted intentionally or not, workers were responsible for reducing racial disparities in the AFST.
For a more in-depth discussion, please read our paper, How Child Welfare Workers Reduce Racial Disparities in Algorithmic Decisions, and our Extended Analysis. All numbers in this blog are from the Extended Analysis. The original paper will be presented at CHI 2022. This work was co-authored with Hao-Fei Cheng, Anna Kawakami, Venkatesh Sivaraman, Yanghuidi Cheng, Diana Qing, Adam Perer, Kenneth Holstein, Steven Wu, and Haiyi Zhu. This work was funded by the National Science Foundation. Also see our concurrent work, Improving Human-AI Partnerships in Child Welfare: Understanding Worker Practices, Challenges, and Desires for Algorithmic Decision Support. We recognize all 48,071 of the children and their families on whom the data in our paper was collected and for whom this data reflects potentially consequential interactions with CYF.