While your TV’s remote might already have a microphone in it for voice commands, it is no replacement for a video store clerk. The current generation of devices respond to a limited set of commands, offer mostly shallow integration with deeper personalization, and may not understand complicated recommendation-seeking questions. Our research aims to develop techniques that can bring together voice recognition technologies, personalization, and advanced search features to provide more natural ways for people to discover new digital content.
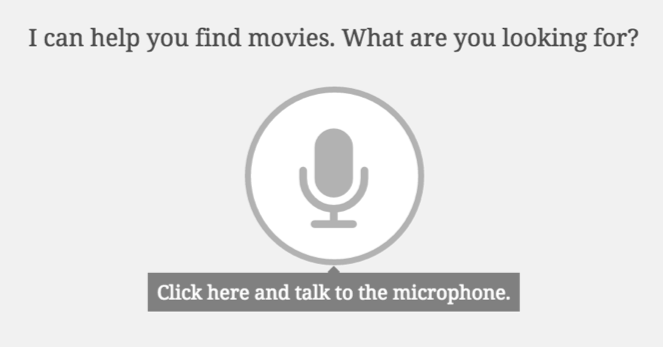
We recently conducted a study to learn more about how people use natural language to look for a movie to watch. We built a prototype system in MovieLens that prompted users with “I can help you find movies. What are you looking for?” The system allowed users to speak or type queries however they wished. We collected and analyzed what they asked for to learn more about typical patterns of use.
We learned that there are several important ways in which people use language differently in the context of recommendation-seeking, as compared with earlier studies of behavior with search engines. We found that people asked for subjective aspects of movies (e.g., “movies with great acting”), but current search techniques have difficulty assessing the relevance between “great acting” and movies. We also found that many people asked for “deep features” (e.g., “movies with open endings”), which are uncommonly tracked in movie databases. These findings indicate a need for new search techniques that can support the full range of what people naturally ask for.
Our research paper from RecSys 2017, Understanding How People Use Natural Language to Ask for Recommendations, includes many more details, including discussion of the differences between speaking and typing queries, and how users ask for better results. We also released a dataset that contains the data we collected in this experiment — we hope this is useful to the research community!
Links: